bstract:
Machine learning has been highly successful in data-intensive applications, but is often hampered when the data set is small. Recently, Few-Shot Learning (FSL) has been proposed to tackle this problem. Using prior knowledge, FSL can rapidly generalize to new tasks containing only a few samples with supervised information. However, the feature representations of the few-shot classes are often biased due to data scarcity. To mitigate this issue, we propose to generate visual samples based on semantic embeddings using a conditional variational autoencoder (CVAE) model. We train this CVAE model on base classes and use it to generate features for novel classes. More importantly, we guide this VAE to strictly generate representative samples by removing non-representative samples from the base training set when training the CVAE model. We show that this training scheme enhances the representativeness of the generated samples and therefore, improves the few-shot classification results. Experimental results show that our method improves three FSL baseline methods by substantial margins, achieving state-of-the-art few-shot classification performance on miniImageNet and tieredImageNet datasets for both 1-shot and 5-shot settings.
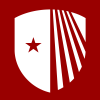