Saad Nadeem’s defense on Thursday, August 3rd, 11am-12noon, Room NCS 120.
Title: Mapping Analytics for Medical Imaging
Abstract:
The prevalence of medical imaging techniques such as computed tomography (CT), magnetic resonance imaging (MRI), and endoscopy has led to intricate and detailed snapshots of human organs. These organs, constituting some of the most complex geometries experienced in the natural world, are difficult to study directly on the imaging data due to their intricate geometry. In this dissertation, a variety of robust mapping techniques are presented to support the visual and analytic analyses of such organs. Novel global and local geometry-mapping techniques are introduced for easier computation, visualization, registration, and segmentation of brain, prostate, pancreas, and colon data. Additionally, novel mappings to abstract representations are presented for more effective medical diagnosis, computer-aided detection, and comparative analysis across patients for colon and lung data.
In the context of global mapping techniques, this dissertation leverages global mesh parameterizations to map entire input mesh/surface/volume data to a canonical domain, such as a plane or a sphere. The resultant spherical and planar mappings are used for creating multimodal brain visualizations, for registering MRI prostate scans, and for registering and visualizing supine-prone virtual colonoscopy (VC) datasets. In case of the colon, haustral fold segmentation and planar angle-preserving mappings are used for registering and visualizing the VC data. For prostate, angle-preserving volumetric spherical mapping is used for registering MRI prostate scans across different modes and time points. Moreover, a novel angle- and area-preserving 3D brain spherical mapping technique is introduced for creating multimodal brain visualizations.
For local geometrical mappings, this dissertation introduces methods for parameterizing and deforming local regions-of-interest in aiding multimodal brain visualization and VC visualization, and for accurately mapping local features-of-interest in brain mesh data and in volumetric scalar data (e.g. CT or MRI). More specifically, a novel local mesh parameterization technique is introduced for conformally deforming and visualizing local regions-of-interest while preserving the overall geometric context on arbitrary surfaces, not possible with the global spherical and planar parameterization techniques. A sulcal pits extraction technique is presented for locally mapping (robust and reproducible) sulcal pits on a given brain mesh using eigenfunction analysis, and an automatic brain parcellation is proposed for locally mapping sulci, gyri and fundi on a brain mesh using a combination of angle- and area-preserving 2D planar mapping, shape measures, and levelsets. A framework is also introduced for locally mapping features-of-interest in volumetric scalar data using prevalent hierarchical segmentation techniques as black-box, and a novel interface for exploring and narrowing down the large number of resultant forest-of-segmentation trees to local features-of-interest.
Finally, abstract representation mappings are used for mediating the task of classifying VC and lung CT data into polyp/polyp-free and nodule/nodule-free regions, respectively, with crowdsourcing. More specifically, novel crowdsourcing studies are designed for polyp and lung nodule detection and annotation. The data collected from these studies is then used in two separate visual analytics frameworks for understanding the anomalies in the crowd and data, which in turn helps in building consensus on the medical diagnosis (classifying polyp/polyp-free and nodule/nodule-free regions). This consensus can help experts speed up the diagnostics and corroborate their findings. Abstract representation mappings are also used for visualizing optical colonoscopy (OC) videos. A computer-aided detection scheme is presented to classify OC video frames into polyp and polyp-free using a novel 3D depth inference technique. Moreover, a visualization framework is introduced for automatic report generation and for comparative OC video analytics.
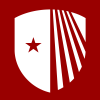