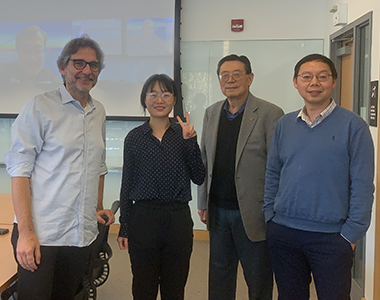
Researchers from Stony Brook University’s Departments of Biomedical Informatics (BMI), Computer Science (CS), and Chemistry recently appeared in Nature Communications< for their work in molecular property prediction.
Professors Fusheng Wang (BMI, CS), Dimitris Samaras (CS), and colleagues authored A Systematic Study of Key Elements Underlying Molecular Property Prediction which was published by Nature Communications October 13, 2023. Within less than a month, the article has been downloaded 5,000 times.
The research examines the use of artificial intelligence (AI) when applied to drug discovery, specifically molecular property prediction. Many would gravitate towards using molecular representation learning as there are plenty of techniques being actively developed for molecular property prediction, which are often evaluated using the MoleculeNet benchmark datasets. However, the study challenges the conventional approach of relying solely on mean and standard deviation for specific performance metrics. While informative, this practice overlooks the crucial element of statistical significance necessary for robust model comparison, revealing that not all newly developed AI techniques unequivocally advance molecular property prediction.
To substantiate this hypothesis, the research team conducted comprehensive experimentation with representative models and datasets with a total of 62,820 trained models. They found that representation learning models show limited performance compared to traditional ML models on fixed representations in most datasets. This led to a further exploration into the reasons behind the occasional failures of representation learning models. Among them, the research team highlighted the importance of dataset size for representation learning models to excel.
Even though the article was recently published, professionals in the field are impressed by their study. The research deepened the understanding and performance of AI-driven drug discovery. One commenter said, “Building on that, Wang and co-workers go one step further and exemplify good ML practice with the widely used MoleculeNet data…Still, the team exposed shortcomings of deep learning algorithms that should dampen unfounded hype around ML…” commented on Wang’s research. (Check out the article commentary here.)
Congratulations to the entire team which also includes Jianyuan Deng (BMI), Zhibo Yang (CS), Hehe Wang (Chemistry) and Iwao Ojima (Chemistry).
-Kimberly Xiao