Xueying Bai will present her work on continual learning with pre-trained models during her thesis proposal defense on Dec 3rd, at 2 pm in NCS 220.
All are welcome. You can also join remotely here: https://stonybrook.zoom.us/j/91241625106?pwd=8TXYpf8460luOSykUQYiQkaJrJqaIO.1
Title: Continual Learning with Pre-trained Language Models
Abstract: Continual Learning (CL) aims to develop models that can sequentially learn from streams of data and tasks, an important need for many real-world applications. One main challenge in CL is to reduce catastrophic forgetting, where models forget knowledge obtained from previous tasks after learning new tasks. In this thesis, we focus on CL with pre-trained language models, which have been shown to significantly benefit learning downstream tasks in NLP. Specifically, we first study factors that can cause interference between gradients of tasks, which may lead to forgetting. We find that this interference depends on hidden representations of models. In response, we first develop a global alignment model that aligns representations across tasks via pre-trained token representations. Then, we study attention patterns in pre-trained LMs and find that an attention sink phenomenon may degrade their CL ability. To address this, we propose a pre-scaling method that encourages models to learn diverse attentions for downstream tasks. These works help to understand the effects of pre-trained models, and better utilize them for the CL purpose. We end with our proposed work that will study redundant solutions and how to utilize them to further improve CL.
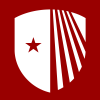