Abstract:Deep learning has been widely adopted in data-intensive clinical applications for diverse medical imaging datasets - from 2D radiographs to 3D magnetic resonance imaging (MRI), computerized tomography (CT) scans, and digital histopathology. However, model performance is often affected in low-resource scenarios such as when dealing with an insufficient number of samples, inadequate access to privileged information during inference, missing modalities during model training and inference, and limited annotated exemplars. Prior approaches are prone to overfitting, struggle to leverage privileged information effectively, fail to train with heterogeneous modality combinations, and are unable to adapt to novel domains without considerable retraining.
This thesis proposal presents several algorithmic innovations to address the aforementioned scenarios: (1) We develop dense feature extraction techniques such as spatio-temporal learning and contrastive learning, coupled with clinically-inspired domain information, to discover meaningful and robust patterns even in small sequential datasets to inject generalizability. (2) We explore recalibration methods that incorporate feature or distribution-matching losses to generate enhanced snapshot representations leveraging the limited temporal representations accessible in training. (3) We employ meta-adversarial learning in latent space to generate modality-agnostic representations that can train with heterogeneous imaging modalities and yet achieve segmentation performance at par with full-modal trained frameworks. (4) We embrace domain generalization via episodic meta-learning, integrating triplet and consistency preserving losses, to design a domain-agnostic segmentation model with limited exemplars. Using these methods, we addressed several unmet clinical needs in radiology (using x-ray, MRI, CT) and digital pathology.
To conclude this thesis, we propose to further investigate the utility of privileged information such as radiologists' eye gaze towards superior medical report generation. The results of this work have been published at MICCAI, CVPR, ICCV, MIDL, etc.
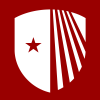